Experimental Design: Types, Examples & Methods
Table Of Content
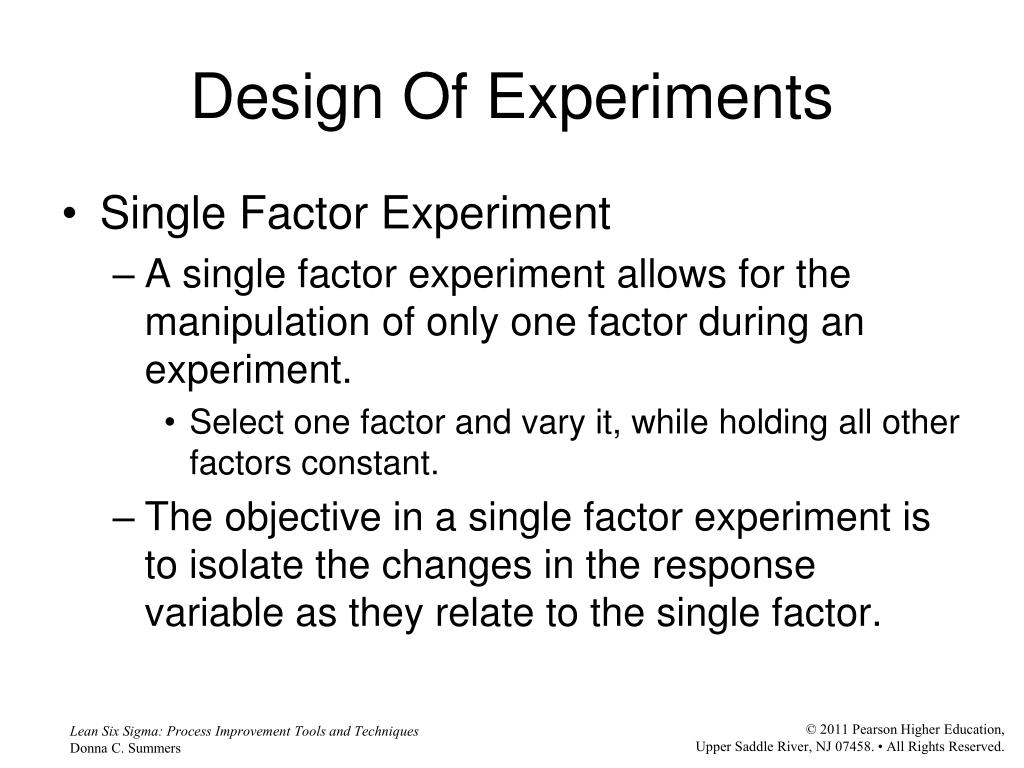
Distinguishing these to inform the next iteration will be critical. Experimental designs are a set of procedures that you plan in order to examine the relationship between variables that interest you. First, you may need to decide how widely to vary your independent variable.
Frequently asked questions about experimental design
Types of design include repeated measures, independent groups, and matched pairs designs. We change the experimental factors and measure the response outcome, which in this case, is the yield of the desired product. Using the COST approach, we can vary just one of the factors at time to see what affect it has on the yield. Within-subjects or repeated measures can also refer to an experimental design where an effect emerges over time, and individual responses are measured over time in order to measure this effect as it emerges.
Bayesian Designs
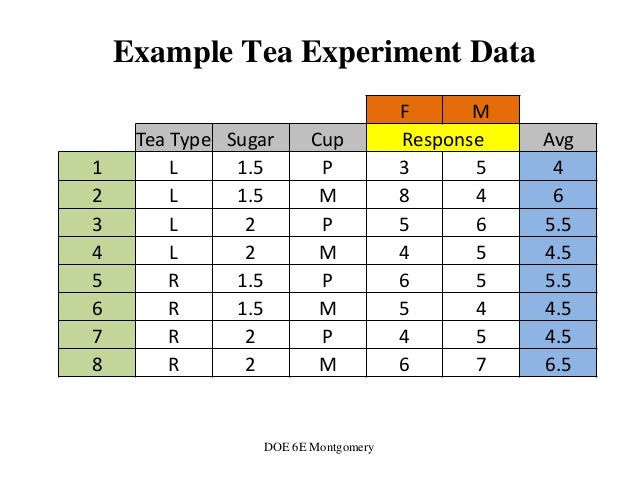
This design's main selling point is its ability to explore interactions between variables. For instance, maybe a new study drug works really well for young people but not so great for older adults. A factorial design could reveal that age is a crucial factor, something you might miss if you only studied the drug's effectiveness in general.
How to reduce errors in Experimental Design?
In planning an experiment, it is necessary to limit any bias that may be introduced by the experimental units or experimental conditions. Strategies such as randomization and blocking can be used to minimize the effect of nuisance or noise elements. Randomly allocating participants to independent variable conditions means that all participants should have an equal chance of taking part in each condition. DOE is about creating an entity of experiments that work together to map an interesting experimental region. So with DOE we can prepare a set of experiments that are optimally placed to bring back as much information as possible about how the factors are influencing the responses. Next, we evaluate what will happen when we fix the volume at 550 ml (the optimal level) and start to change the second factor.
DOE in Fast-Moving Consumer Goods (FMCG) industry
A well-known example of Crossover Design is in studies that look at the effects of different types of diets—like low-carb vs. low-fat diets. Researchers might have participants follow a low-carb diet for a few weeks, then switch them to a low-fat diet. By doing this, they can more accurately measure how each diet affects the same group of people.
How To Add Efficiency From Development To Validation With Design Of Experiments Part 1 - BioProcess Online
How To Add Efficiency From Development To Validation With Design Of Experiments Part 1.
Posted: Fri, 10 Mar 2023 08:00:00 GMT [source]
The fields that get the most out of True Experimental Designs are those that need super reliable results, like medical research.
Covariate Adaptive Randomization
They can be very complex to plan and carry out, and there's always a risk that the changes made during the study could introduce bias or errors. This method is particularly useful in fast-paced or high-stakes situations, like developing a new vaccine in the middle of a pandemic. The ability to adapt can save both time and resources, and more importantly, it can save lives by getting effective treatments out faster. One famous use of Pretest-Posttest Design is in evaluating the effectiveness of driver's education courses. Researchers will measure people's driving skills before and after the course to see if they've improved. It's pretty easy to understand and doesn't require fancy statistics.
Apply Full Factorial DOE on the same example
For example, with eight factors, 256 runs would theoretically be necessary. Fortunately, because three-factor and higher-order interactions are rarely important, such intensive efforts are seldom required. For most purposes, it is only necessary to evaluate the main effects of each variable and the two-factor interactions, which can be done with only a fraction of the runs in a full factorial design. If there are some two-factor interactions that are known to be impossible, one can further reduce the number of runs by using Resolution IV designs. Table V compares the number of runs in full and fractional factorial designs with from two to eight variables. For the earlier example of eight factors, one can create an efficient design that may require as little as 16 runs.
Suppress and reduce impurities in drugs production phase
Carefully planned, statistically designed experiments offer clear advantages over traditional one-factor-at-a-time alternatives. These techniques are particularly useful tools for process validation, where the effects of various factors on the process must be determined. Not only is the DOE concept easily understood, the factorial experiment designs are easy to construct, efficient, and capable of determining interaction effects. Results are easy to interpret and lead to statistically justified conclusions. The designs can be configured to block out extraneous factors or expanded to cover response surface plotting.
Meet the Cluster Randomized Design, the team captain of group-focused research. In our imaginary lineup of experimental designs, if other designs focus on individual players, then Cluster Randomized Design is looking at how the entire team functions. Its major strength is in reducing the "noise" that comes from individual differences.
It helps to manage process inputs in order to optimize the output. In a within-subjects design, each participant experiences all conditions, and researchers test the same participants repeatedly for differences between conditions. In a between-subjects design, every participant experiences only one condition, and researchers assess group differences between participants in various conditions.
The first column in the table shows the run number for the four possible runs. Columns 5 and 6 show the actual values assigned to the low and high variable levels in the design. Test runs using each of these four combinations constitute the experiment. The last column contains the responses from the experiment, which in Table II are the data from Figure 2. Filling in this column requires the hard work of running each experiment and then recording the result.
Just like you wouldn't start building without a good blueprint, researchers won't start their studies without a strong experimental design. Alright, before we dive into the different types of experimental designs, let's get crystal clear on what experimental design actually is. Since only the ABC effect changed by the magnitude of the difference between batches, the batch-to-batch difference had been successfully removed from the experiment by its inclusion in the experimental setup. Without this blocking, the determination of the variables' effects would have been less precise, or missed altogether. It is well recognized that the planning activities that precede the actual test runs are critical to the successful resolution of the experimenter's problem.
Comments
Post a Comment