Design of Experiments Blocking and Full Factorial Experimental Design Plans
Table Of Content
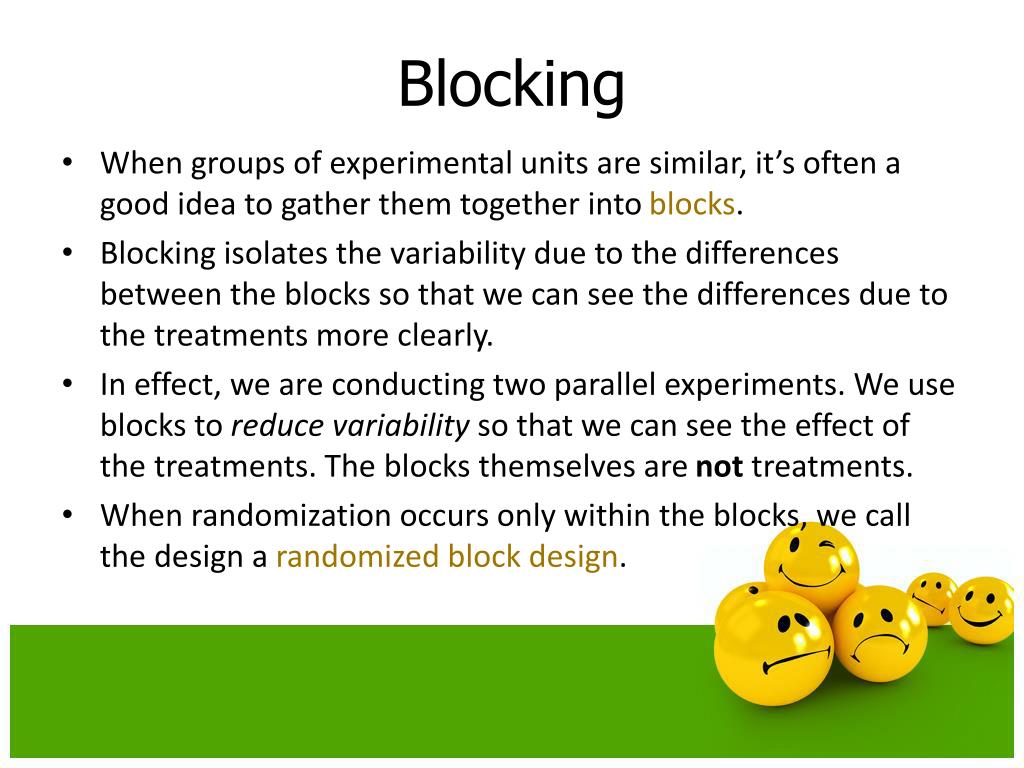
If it only means order and all the cows start lactating at the same time it might mean the same. But if some of the cows are done in the spring and others are done in the fall or summer, then the period effect has more meaning than simply the order. Although this represents order it may also involve other effects you need to be aware of this. A Case 3 approach involves estimating separate period effects within each square. The following crossover design, is based on two orthogonal Latin squares.
Table of Contents
Here are some examples of what your blocking factor might look like. By extension, note that the trials for any K-factor randomized block design are simply the cell indices of a k dimensional matrix. After I promised an introduction to blocking in design of experiments last time, one of our Minitab statisticians came to see me.
Why is the randomized controlled double-blind experiment ideal?
We do not have observations in all combinations of rows, columns, and treatments since the design is based on the Latin square. For an odd number of treatments, e.g. 3, 5, 7, etc., it requires two orthogonal Latin squares in order to achieve this level of balance. For even number of treatments, 4, 6, etc., you can accomplish this with a single square.
About
Design and realization of data mining simulation and methodological models - ScienceDirect.com
Design and realization of data mining simulation and methodological models.
Posted: Wed, 01 Nov 2023 15:14:45 GMT [source]
What you also want to notice is the standard error of these means, i.e., the S.E., for the second treatment is slightly larger. The fact that you are missing a point is reflected in the estimate of error. You do not have as many data points on that particular treatment. Basic residual plots indicate that normality, constant variance assumptions are satisfied. Therefore, there seems to be no obvious problems with randomization. These plots provide more information about the constant variance assumption, and can reveal possible outliers.
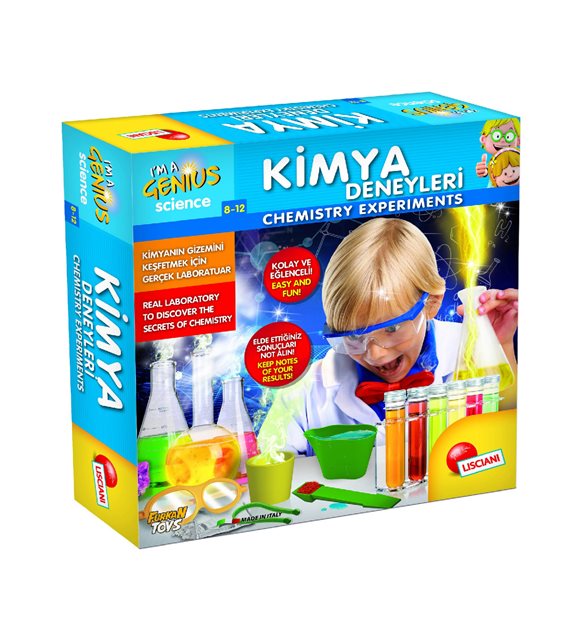
Therefore we partition our subjects by gender and from there into age classes. Thus we have a block of subjects that is defined by the combination of factors, gender and age class. The final step in the blocking process is allocating your observations into different treatment groups. All you have to do is go through your blocks one by one and randomly assign observations from each block to treatment groups in a way such that each treatment group gets a similar number of observations from each block. The first step of implementing blocking is deciding what variables you need to balance across your treatment groups.
4: Blocking in 2 Dimensions - Latin Square
In this case, we have different levels of both the row and the column factors. Again, in our factory scenario, we would have different machines and different operators in the three replicates. In other words, both of these factors would be nested within the replicates of the experiment. For instance, we might do this experiment all in the same factory using the same machines and the same operators for these machines. The first replicate would occur during the first week, the second replicate would occur during the second week, etc.
Because the specific details of how blocking is implemented can vary a lot from one experiment to another. For that reason, we will start off our discussion of blocking by focusing on the main goal of blocking and leave the specific implementation details for later. You can see this by these contrasts - the comparison between block 1 and Block 2 is the same comparison as the AB contrast.
About The Author
The fundamental idea of blocking can be extended to more dimensions. However, the full use of multiple blocking variables in a complete block design usually requires many experimental units. Latin Square design can be useful when we want to achieve blocking simultaneously in two directions with a limited number of experimental units.
Sorry, you have been blocked
Randomization, Blocking, and Re-Randomization by Leihua Ye, PhD - Towards Data Science
Randomization, Blocking, and Re-Randomization by Leihua Ye, PhD.
Posted: Sun, 21 Nov 2021 08:00:00 GMT [source]
The aim is to support basic data science literacy to all through clear, understandable lessons, real-world examples, and support. Here is an alternative way to analyze this design using the analysis portion of the fractional factorial software in Minitab v.16. Minitab’s General Linear Command handles random factors appropriately as long as you are careful to select which factors are fixed and which are random. Switch them around...now first fit treatments and then the blocks. The sequential sums of squares (Seq SS) for block is not the same as the Adj SS. Let's take a look at how this is implemented in Minitab using GLM.
All ordered pairs occur an equal number of times in this design. It is balanced in terms of residual effects, or carryover effects. Together, you can see that going down the columns every pairwise sequence occurs twice, AB, BC, CA, AC, BA, CB going down the columns. The combination of these two Latin squares gives us this additional level of balance in the design, than if we had simply taken the standard Latin square and duplicated it. Crossover designs use the same experimental unit for multiple treatments. The common use of this design is where you have subjects (human or animal) on which you want to test a set of drugs -- this is a common situation in clinical trials for examining drugs.
Another way to think about this is that a complete replicate of the basic experiment is conducted in each block. In this case, a block represents an experimental-wide restriction on randomization. Back to the hardness testing example, the experimenter may very well want to test the tips across specimens of various hardness levels. To conduct this experiment as a RCBD, we assign all 4 tips to each specimen. Sometimes several sources of variation are combined to define the block, so the block becomes an aggregate variable. Consider a scenario where we want to test various subjects with different treatments.
If you think a variable could influence the response, you should block on that variable. Here is a plot of the least square means for treatment and period. We can see in the table below that the other blocking factor, cow, is also highly significant.
Comments
Post a Comment